The advent of artificial intelligence (AI) has revolutionized numerous aspects of modern life, and one of the most significant impacts has been on research efficiency. By automating repetitive tasks, analyzing vast amounts of data, and providing insights that would be impossible for humans to achieve alone, AI has become an indispensable tool in the research landscape. From academia to industry, researchers are leveraging AI to streamline their processes, enhance the quality of their work, and accelerate the discovery of new knowledge.
The integration of AI in research has been particularly noteworthy in fields such as biology, physics, and social sciences, where the sheer volume of data can be overwhelming. For instance, in genomic research, AI algorithms can analyze thousands of genetic sequences in a fraction of the time it would take human researchers, identifying patterns and correlations that could lead to breakthroughs in disease diagnosis and treatment. Similarly, in astrophysics, AI can sift through vast amounts of observational data from telescopes and satellites, helping scientists to identify new celestial objects and phenomena that would otherwise go unnoticed.
Key Points
- AI automates repetitive research tasks, freeing up time for more strategic and creative work.
- AI analyzes vast amounts of data, providing insights that would be impossible for humans to achieve alone.
- AI-enhanced research leads to faster discovery and publication of new findings.
- AI facilitates collaboration among researchers from diverse disciplines, fostering a more interdisciplinary approach to science.
- AI raises important ethical considerations, including issues related to data privacy, bias, and accountability.
Accelerating Discovery with AI

One of the most significant benefits of AI in research is its ability to accelerate the discovery process. By analyzing large datasets and identifying patterns, AI can help researchers to formulate hypotheses, design experiments, and test theories more efficiently. For example, in materials science, AI can predict the properties of new materials and suggest optimal synthesis routes, reducing the need for trial-and-error experimentation. This not only saves time and resources but also enables researchers to explore a wider range of possibilities, leading to more innovative and groundbreaking discoveries.
AI-Powered Data Analysis
AI’s ability to analyze vast amounts of data is particularly valuable in fields where data is abundant but complex. For instance, in climate science, AI can analyze satellite imagery, sensor data, and climate models to predict weather patterns, track changes in sea level, and identify areas of high conservation value. This information can be used to inform policy decisions, develop more effective conservation strategies, and mitigate the impacts of climate change. Furthermore, AI can help researchers to identify biases in datasets and develop more robust methods for data analysis, leading to more reliable and generalizable findings.
Research Field | AI Application | Benefits |
---|---|---|
Genomics | Genetic sequence analysis | Identification of disease-causing genes, development of personalized medicine |
Astrophysics | Observational data analysis | Discovery of new celestial objects, understanding of cosmic phenomena |
Materials Science | Prediction of material properties | Development of new materials, optimization of synthesis routes |
Climate Science | Analysis of satellite imagery and sensor data | Prediction of weather patterns, tracking of climate change impacts |
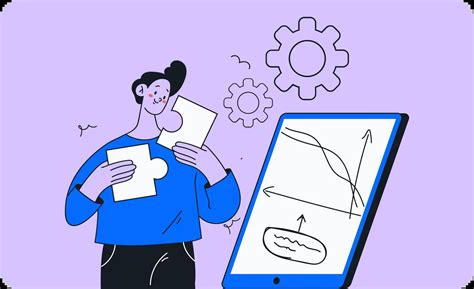
Challenges and Limitations of AI in Research
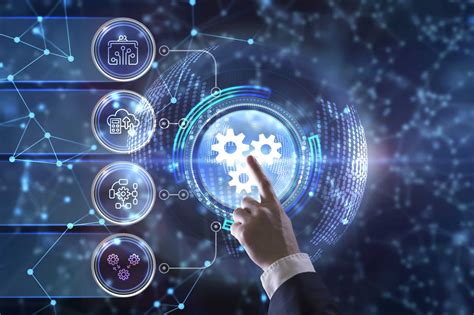
While AI has the potential to greatly enhance research efficiency, there are also challenges and limitations to its adoption. One of the primary concerns is the issue of bias in AI algorithms, which can perpetuate existing inequalities and lead to flawed conclusions. Furthermore, the lack of transparency and explainability in AI decision-making processes can make it difficult for researchers to understand and trust the results. Additionally, the reliance on high-quality data and the need for significant computational resources can be barriers to entry for some researchers, particularly those in under-resourced institutions or from underrepresented groups.
Addressing the Challenges of AI in Research
To fully realize the benefits of AI in research, it is essential to address these challenges and limitations. This can be achieved through the development of more transparent and explainable AI algorithms, the creation of diverse and representative datasets, and the provision of training and support for researchers to develop the necessary skills to work with AI. Furthermore, researchers must be aware of the potential biases and limitations of AI and take steps to mitigate them, such as using multiple sources of data and validating results through human oversight and peer review.
What are the primary benefits of using AI in research?
+The primary benefits of using AI in research include the ability to automate repetitive tasks, analyze vast amounts of data, and provide insights that would be impossible for humans to achieve alone. AI can also facilitate collaboration among researchers, accelerate the discovery process, and lead to more innovative and groundbreaking findings.
What are the challenges and limitations of AI in research?
+The challenges and limitations of AI in research include the issue of bias in AI algorithms, the lack of transparency and explainability in AI decision-making processes, and the reliance on high-quality data and significant computational resources. Additionally, there are concerns about the potential displacement of human researchers and the need for ongoing training and support to develop the necessary skills to work with AI.
How can researchers address the challenges and limitations of AI in research?
+Researchers can address the challenges and limitations of AI in research by developing more transparent and explainable AI algorithms, creating diverse and representative datasets, and providing training and support for researchers to develop the necessary skills to work with AI. Additionally, researchers must be aware of the potential biases and limitations of AI and take steps to mitigate them, such as using multiple sources of data and validating results through human oversight and peer review.
In conclusion, AI has the potential to greatly enhance research efficiency, accelerating the discovery process and leading to more innovative and groundbreaking findings. However, it is essential to address the challenges and limitations of AI in research, including the issue of bias, the lack of transparency and explainability, and the reliance on high-quality data and significant computational resources. By developing more transparent and explainable AI algorithms, creating diverse and representative datasets, and providing training and support for researchers, we can unlock the full potential of AI in research and drive progress in our respective fields.